Goals as Reward-Producing Programs
Feb 1, 2025·,,,,·
1 min read
Guy Davidson
Graham Todd
Julian Togelius
Todd M. Gureckis
Brenden M. Lake
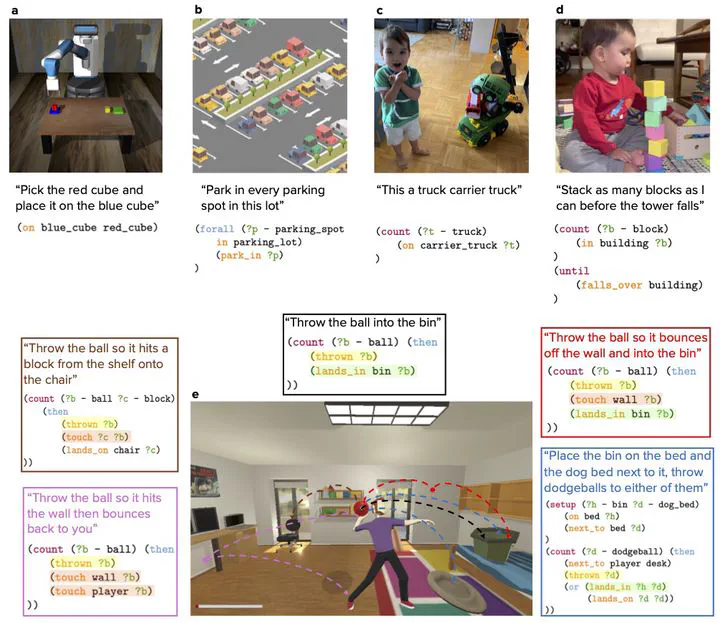
Abstract
People have a remarkable capacity to generate their own goals, beginning with child’s play and continuing into adulthood. Despite considerable empirical and computational work on goals and goal-oriented behavior, models are still far from capturing the richness of everyday human goals. Here we bridge this gap by collecting a dataset of human-generated playful goals, modeling them as reward-producing programs, and generating novel human-like goals through program synthesis. Reward-producing programs capture the rich semantics of goals through symbolic operations that compose and add temporal constraints, and allow for program execution on behavioral traces to evaluate progress. To build a generative model of goals, we learned a fitness function over the infinite set of possible goal programs, and sample novel goals with a quality-diversity algorithm. Human evaluators found the model’s better samples indistinguishable from human-created games. We also discovered that our model’s internal fitness scores predict games that are evaluated as more fun to play and more human-like.
Type
Publication
Nature Machine Intelligence
Add the full text or supplementary notes for the publication here using Markdown formatting.